

Scalable AI Incident Classification
A number of AI incident databases exist, but their data is inconsistently structured. I’ve developed a tool to structure AI incident data and presented the results with a dashboard, to help policymakers make evidence-based decisions. The tool classifies real-world, reported incidents by risk category and harm caused, aiming to reveal information about the impacts of AI on society that is currently opaque.
Using an LLM, the tool processes raw incident reports, providing a scalable methodology that could be applied cost-effectively across much larger datasets as numbers of reported incidents grow.
I classified all incidents in the AI Incident Database according to the MIT Risk Repository’s causal and domain taxonomies. I then assigned scores for harm-severity on 10 different dimensions based on the CSET AI Harm Taxonomy, using a scale I developed to reflect impact from zero to 'worst-case catastrophe'.
The outputs are a structured dataset and a dashboard that you can explore through a graphical web interface to identify trends and insights.
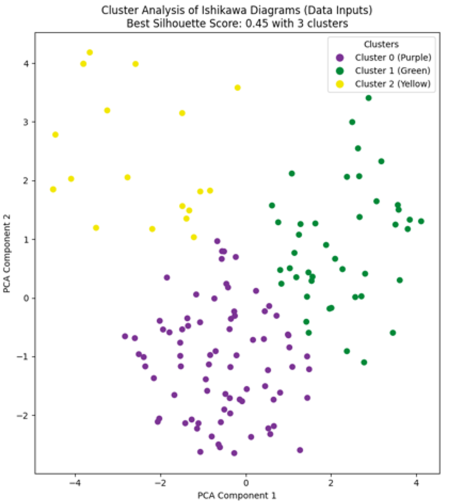
Validity and Clustering of AI Safety Incident Causality Analysis
This article identifies and addresses some shortcomings and potential objections to the original Root Cause Analysis method including reliability and epistemology of responses.
It then describes a cluster analysis of 50 incident reports aimed at identifying correlations between clusters of causes and different types of harm.
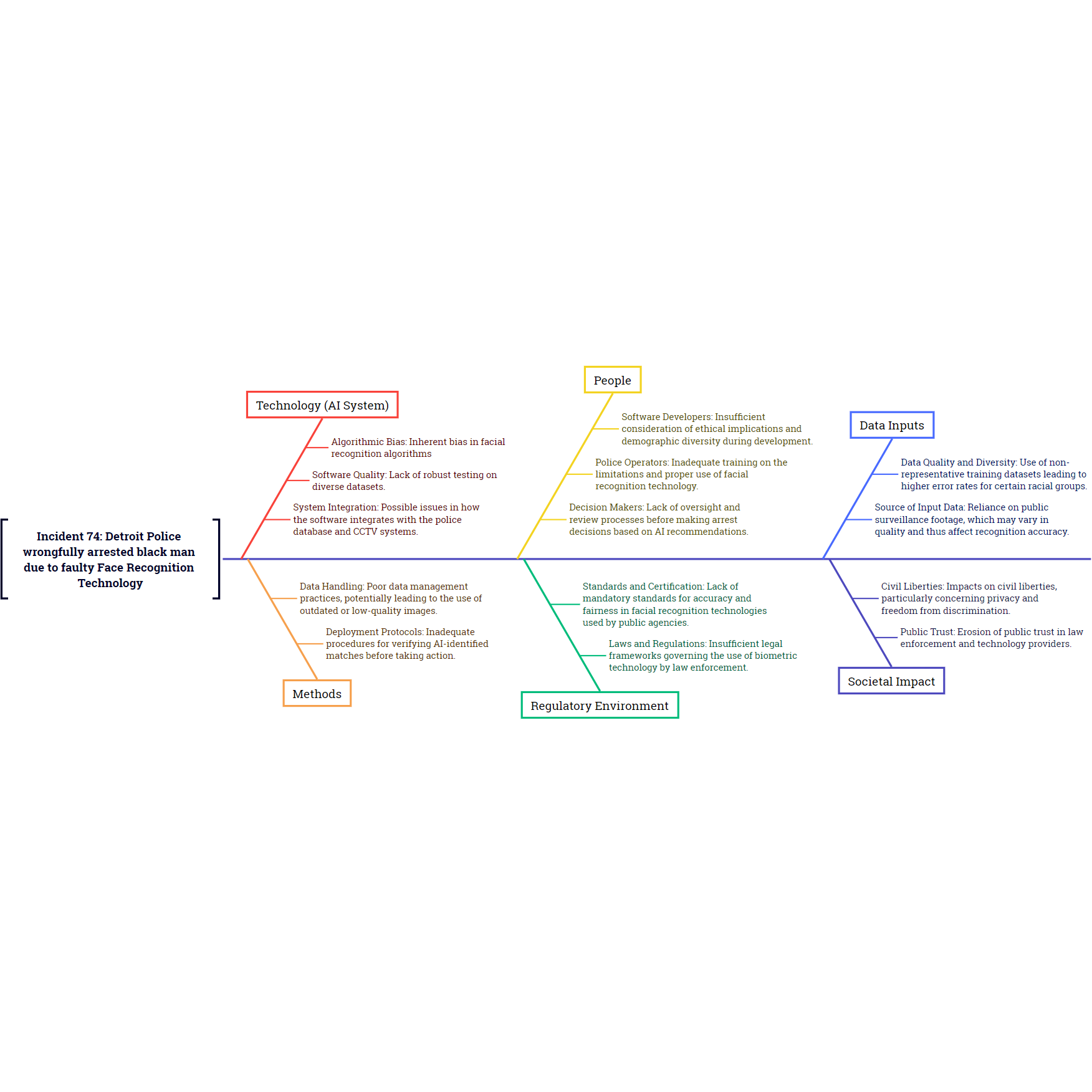
Root Cause Analysis of AI Safety Incidents
The application of Root Cause Analysis tools that are widely used in other areas of engineering could provide insights into the causes of AI safety incidents that would reduce the likelihood of recurrence and support the prioritisation of risk mitigation and harm reduction measures.
This post proposes and demonstrates an approach for using language models to process incident reports and make inferences as to potential causes.
The approach could offer a scalable methodology to aggregate causality data from historic incidents and potentially from modelled future scenarios, in order to contribute to a reduction in the numbers of future safety incidents and the severity of harm caused by them.